
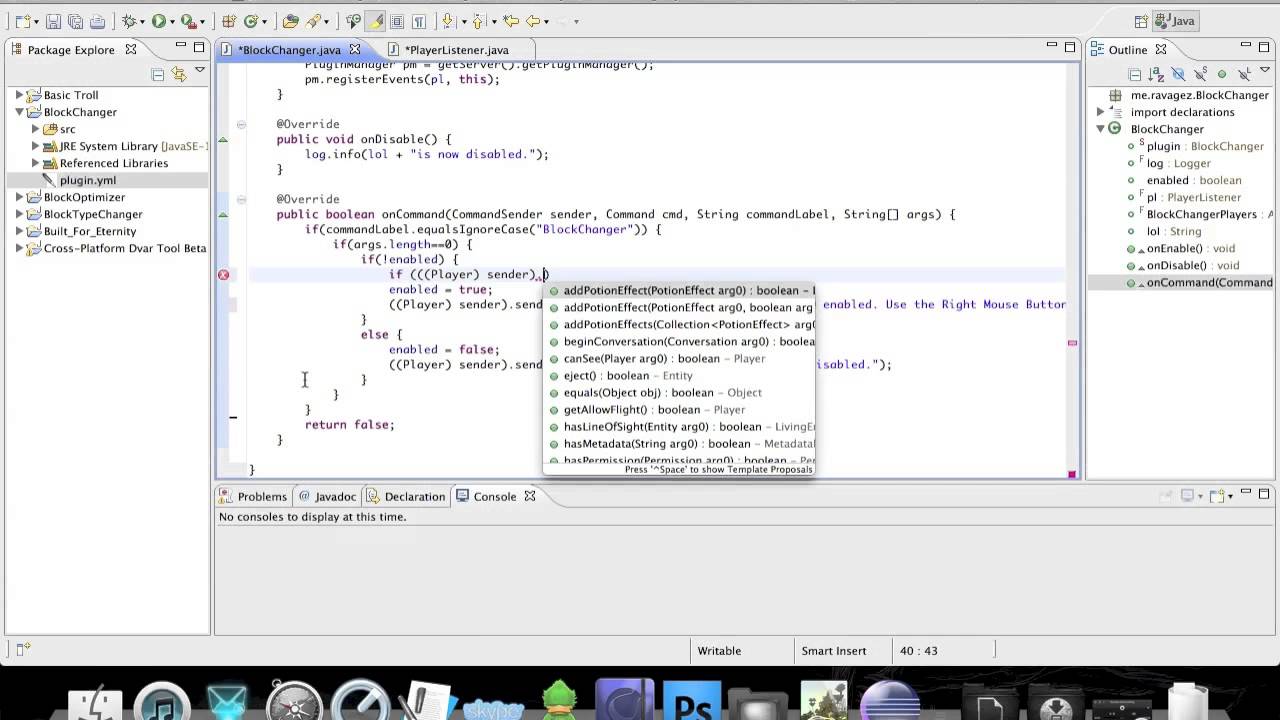
Comment: 160+ pages, doctoral dissertation (latex with figures) In experiments between a parser, acquired using this statistical framework, and a grammarian's rule-based parser, developed over a ten-year period, both using the same training material and test sentences, the decision tree parser significantly outperformed the grammar-based parser on the accuracy measure which the grammarian was trying to maximize, achieving an accuracy of 78% compared to the grammar-based parser's 69%. By basing the disambiguation criteria selection on entropy reduction rather than human intuition, this parser development method is able to consider more sentences than a human grammarian can when making individual disambiguation rules. These decision trees take advantage of significant amount of contextual information, potentially including all of the lexical information in the sentence, to produce highly accurate statistical models of the disambiguation process. Based on distributionally-derived and linguistically-based features of language, this parser acquires a set of statistical decision trees which assign a probability distribution on the space of parse trees given the input sentence.
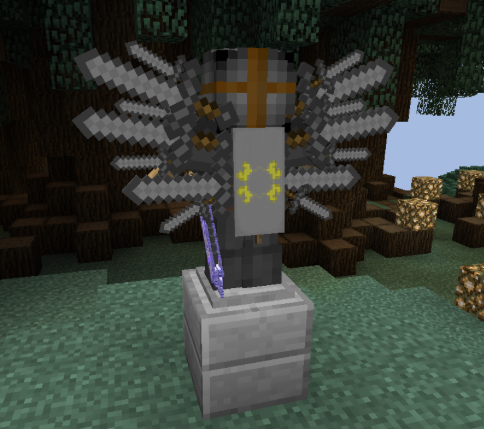
In this work, I propose an automatic method for acquiring a statistical parser from a set of parsed sentences which takes advantage of some initial linguistic input, but avoids the pitfalls of the iterative and seemingly endless grammar development process. A majority of the grammarian's efforts are devoted to the disambiguation process, first hypothesizing rules which dictate constituent categories and relationships among words in ambiguous sentences, and then seeking exceptions and corrections to these rules. Traditional natural language parsers are based on rewrite rule systems developed in an arduous, time-consuming manner by grammarians. In our third work, we obtain state-of-the-art results with our unsupervised dependency parser for eight different lan- guages. In the first two approaches concern- ing supervised phrase structure parsing, we improve the performance of two state-of-the-art parsers. The results we obtain with our three different approaches look promising. In addition, our experiments with these grammars gave us practical understanding about their properties.įrom this three works we see that by using automata we can model syntac- tic structures for different purposes. As Bilexical Grammars are based on finite automata, we inherit their good learnabil- ity properties. We use the formalism of Bilexical Grammars as a way to model syntac- tical structures of sentences throughout our whole thesis. In the third approach, we go a step further and propose an architecture for building multi-language unsupervised parsers that can learn structures based just on samples of data. Namely, the construction of Part-of-Speech tag sets and the finding of heads of syntactic constituents. In two of them, we employ Genetic Algorithms to automatically infer data driven solutions to problems which were treated manually in previous works. We present different approaches where we apply computational methods to NLP. This thesis is concerned with different ways to benefit from the techno- logical possibility to refine and justify former knowledge based on more rationalistic treatments of Syntax in Natural Language Processing (NLP). Nowadays we can test and derive hypotheses from the automatic processing of the huge amount of digital- ized texts. When computers appeared and large quantities of data could be processed a new, more empirical, ap- proach to the study of languages arose. Natural languages phenomena have been studied by linguistic tradition well before the invention of computers.
